文章目錄
-
* 一、關於基金定投
-
* 數據來源
- 接口規范
- 常見指數基金/股票代碼
- 二、分析目標
- 三、代碼實現
-
* 1、定義獲取數據、清洗數據的函數
- 2、定義定投策略函數
- 3、計算2019年對滬深300指數基金進行定投的收益率
- 4、假設定投的金額是500元,每周定投一次,據此分別計算從2002年開始到2019年,每年定投滬深300指數基金的收益率並將結果可視化
- 5、實現"慧定投"(支付寶中的智能定投策略)
- 定義定投策略(對於每個交易日前一日的收盤價)
- 四、可視化普通定投與智能定投策略收益率
- 五、文末福利(完整代碼)
-
基於Python的基金定投分析
通過獲取過去10年的股市數據分析基金定投的收益率。
一、關於基金定投
基金定投,就是按照固定的頻率和固定的金額,不停分批次小額買入金融市場上的基金。為什么要這么麻煩,這樣做有什么好處嗎?我們本次“數據分析和挖掘”的第四次作業就
指數基金定投 的效果來分析一下。
注:定投可以選擇任何種類的基金。我們以指數基金為例,因為大部分傳說中 無腦定投 的案例都是以指數基金的定投方式
數據來源
- 通過API接口從網易財經上進行調取
接口規范
其中
- code參數后面的7位整數代表了股票代碼;比如0000001指的是上證指數。注意這串數字要分0和000001兩部分看。0代表sh,1代表sz。所以0000001其實是sh000001的意思。同理,0 000300也就是sh000300 滬深300的代碼。
- start和end參數后面的8位整數代表年(xxxx)月(xx)日(xx)
- fields選項中,TCLOSE,HIGH,LOW,TOPEN分別表示當日的收盤,最高,最低,開盤價;LCLOSE表示昨日收盤價。CHG,PCHG,VOTURNOVER,VAT分別表示漲跌額,漲跌幅,成交量,成交金額。
常見指數基金/股票代碼
- 0000300 - 滬深300
- 0000001 - 上證指數
- 0000016 - 上證50
- 399005 - 中小板指
- 399673 - 創業板50
- 000903 - 中證100
二、分析目標
- 假設定投的金額是500元,每周定投一次,據此計算2019年對 滬深300指數 基金進行定投的收益率
- 假設定投的金額是500元,每周定投一次,據此 分別 計算從2002年開始到2019年,每年定投 滬深300指數基金 的收益率並將結果可視化
- 探索不同的定投策略。這里以支付寶的“慧定投”智能定投策略為例
注:不同的定投策略可以是改變定投周期(比如從每周定投到每月定投),改變定投金額(比如從固定金額到固定比例),設定止損或者止盈策略等等。
三、代碼實現
1、定義獲取數據、清洗數據的函數
import pandas as pd
import numpy as np
import matplotlib.pyplot as plt
import requests
import warnings
warnings.filterwarnings('ignore')
plt.rcParams['font.family']='SimHei'
plt.rcParams['axes.unicode_minus']=False
plt.rcParams['figure.figsize']=(12,8)
def get_data(code,start,end):
'''
獲取指定時間范圍的股票數據
:param code: 指數代碼
:param start: 起始日期
:param end: 截止日期
:return: DataFrame
'''
url='http://quotes.money.163.com/service/chddata.html?code={}&start={}&end={}&fields=TCLOSE;HIGH;LOW;TOPEN;LCLOSE;CHG;PCHG;VOTURNOVER;VATURNOVER'.format(code,start,end)
name=code+'_'+start+"-"+end
f=open(name,'wb')
f.write(requests.get(url).content)
f.close()
data=pd.read_csv(name,encoding='gbk')
return data
def clean_data(data):
'''
1、將日期轉換為時間序列並設置為索引
2、將數據按時間序列升序排序
3、刪除缺失值
4、將漲跌幅的單位轉換為小數
'''
data['日期']=pd.to_datetime(data['日期'])
data=data.set_index("日期")
data=data.sort_index()
data.drop(data[data["漲跌幅"]=="None"].index,axis=0,inplace=True)
data["漲跌幅"]=data['漲跌幅'].astype('float')
data['漲跌幅']/=100
return data
獲取滬深300的數據,並查看
df1=get_data('0000300','20190102','20191231')
df1=clean_data(df1)
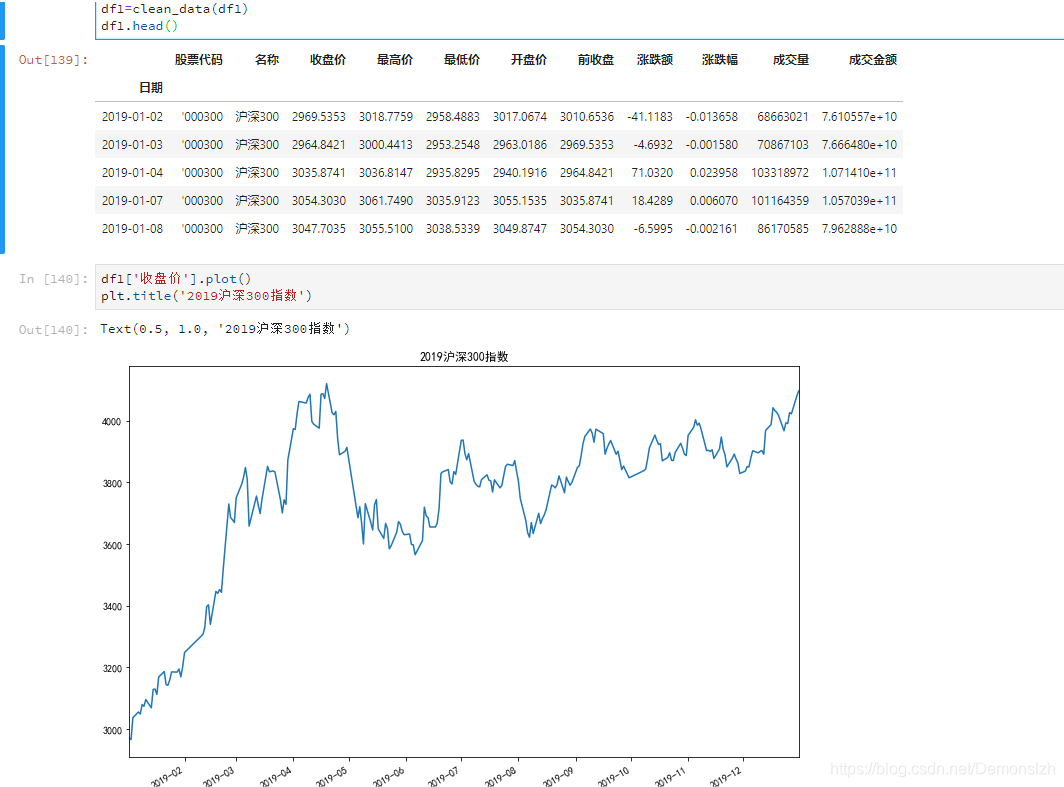
2、定義定投策略函數
def invest(df1,frequence,invest_money,start_time):
'''
定投計算
:param df1: 數據集
:param frequence: 定投頻率
:param invest_money: 每次定投金額
:param start: 定投起始日期
:return (amount,invest_log): (收益數據DataFrame,定投記錄dict)
'''
invest_log={}#每次定投的日期記錄(日期:大盤指數)
invest_day=start_time#每次投資的時間
invest_amount=0#總投資金額
profile=0#總投資收益
amount=0#賬戶總資產
profile_log=[]#總收益日志
invest_amount_log=[]#賬戶投資金額日志
amount_log=[]#總資產日志
Yield=[]#收益率日志
for date,quote_change,index in zip(df1.index,df1['漲跌幅'],df1['收盤價']):
profile+=quote_change*amount#計算當天收益率
profile_log.append(profile)
#判斷是否為定投日
if date==invest_day:
invest_amount+=invest_money
invest_log[invest_day]=index#記錄定投當日的指數
#判斷7天后是否為交易日,如果不是則往后加1天直到找到交易日
invest_day+=np.timedelta64(frequence,'D')
flag=0
while(True):
if(df1[df1.index==invest_day].index==invest_day):
break
else:
invest_day+=np.timedelta64(1,'D')
flag+=1
if(flag==100):
break
invest_amount_log.append(invest_amount)
amount=invest_amount+profile#更新賬戶總資產
amount_log.append(amount)
try:
Yield.append(profile/invest_amount*100)#更新收益率
except:
Yield.append(0)
print("總投資:",invest_amount)
print("總收益:",profile)
print("收益率: ",profile/invest_amount*100,"%")
over=pd.DataFrame({
"日期":df1.index,
"收益率":Yield,
"賬戶資產":amount_log,
"投資金額":invest_amount_log
})
over=over.set_index("日期")
return over,invest_log
3、計算2019年對滬深300指數基金進行定投的收益率
frequence=7#定投頻率
invest_money=500#每次定投金額
start=np.datetime64("2019-01-02")
print("2019年定投結果顯示:")
res,buy=invest(df1,frequence,invest_money,start)
def myplot(df1,res,buy,titlename):
'''
繪制定投結果圖
'''
plt.figure()
df1['收盤價'].plot(label="大盤指數")
plt.scatter(buy.keys(),buy.values(),color="brown",marker=".",label="定投記錄")
plt.legend(loc='best')
plt.ylabel("指數")
plt.twinx()
res['賬戶資產'].plot(color="red")
res['投資金額'].plot(color="orange")
plt.ylabel("元")
plt.legend()
plt.title(titlename+":{:.2f}%".format(res.tail(1)["收益率"][0]))
plt.show()
titlename="2019年滬深300定投收益率"
myplot(df1,res,buy,titlename)
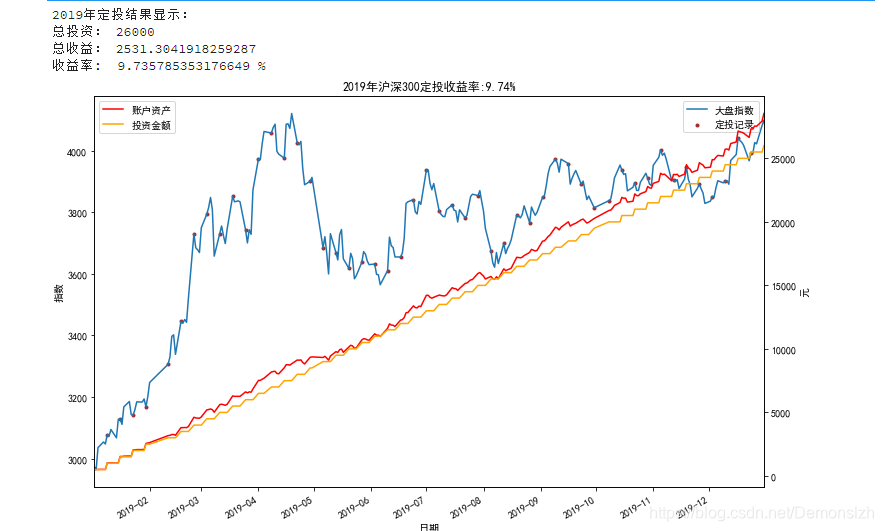
4、假設定投的金額是500元,每周定投一次,據此分別計算從2002年開始到2019年,每年定投滬深300指數基金的收益率並將結果可視化
df2=get_data('0000300','20020101','20191231')
df2=clean_data(df2)
df2['收盤價'].plot()
plt.title("2002年-2019年滬深300指數")
frequence=7#定投頻率
invest_money=500#每次定投金額
print("從2002年開始定投到2019年結束的收益情況:")
start=np.datetime64("2002-01-07")
res,buy=invest(df2,frequence,invest_money,start)
x=[str(i) for i in range(2002,2020)]
y=[]
for year in range(2002,2020):
'''每年的收益率:年末收益率-年初收益率'''
each_year=res[res.index.year==year].tail(1)["收益率"][0]-res[res.index.year==year].head(1)["收益率"][0]
y.append(each_year)
plt.title("2002年-2019年 滬深300 年定投收益率")
plt.ylabel("收益率(%)")
plt.xlabel("年")
plt.plot(x,y,label="年收益率")
plt.plot(x,[res.tail(1)["收益率"][0] for i in range(len(x))],ls="--",alpha=0.5,label="總投資收益率")
plt.plot(x,[0 for i in range(len(x))],color="gray",ls="--",alpha=0.3)
plt.xlim("2002","2019")
plt.legend()
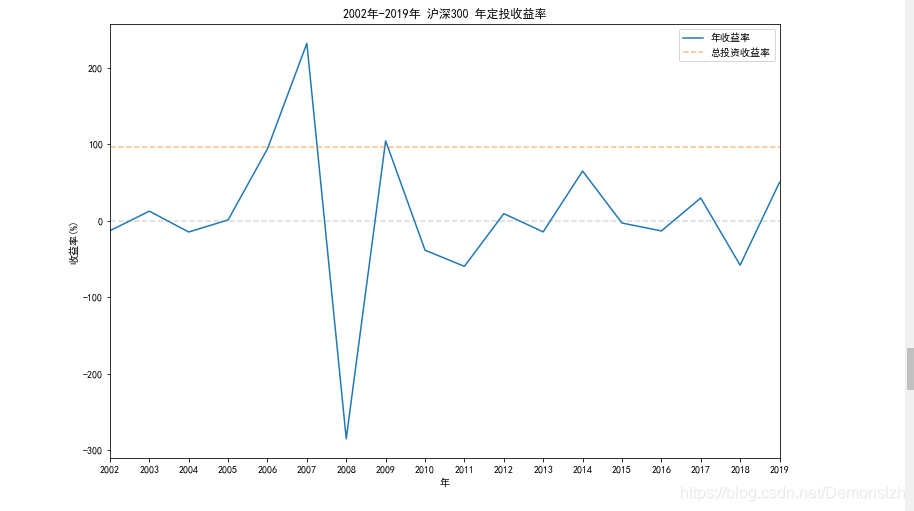
5、實現"慧定投"(支付寶中的智能定投策略)
定義定投策略(對於每個交易日前一日的收盤價)
- 高於均線15%以內,扣款90%
- 高於均線15-50%,扣款80%
- 高於均線50-100% 扣款70%
- 高於均線 100%以上 扣款60%
-
近10日振幅小於5%
- 低於均線5%以內,扣款180%
- 低於均線5-10%以內,扣款190%
- 低於均線10-20%以內,扣款200%
- 低於均線20-30%以內,扣款210%
- 低於均線30-40%以內,扣款220%
- 低於均線40%以上,扣款230%
-
近10日振幅大於5%
- 低於均線5%以內,扣款60%
- 低於均線5-10%以內,扣款70%
- 低於均線10-20%以內,扣款80%
- 低於均線20-30%以內,扣款90%
- 低於均線30-40%以內,扣款100%
- 低於均線40%以上,扣款110%
所以在這個過程中我們需要定義幾個函數,由於要考慮到均線與振幅的問題,所以首先需要定義均線、振幅函數,獲取基金n日收益與m日振幅
def mean_days(df,days,wave):
'''
獲取n日均線,最近m日振幅
:param df: 數據集
:param days: 均線天數
:params wave:最近m日振幅
:return average,wavelength:均線、近m日振幅
'''
average=[]
wavelength=[]
start=df.head(1).index-np.timedelta64(days+1,"D")
start=start.astype('str')[0].replace("-","")#轉換為字符串
df4=get_data('0000300',start,'20191231')#獲得原始數據前days天的數據用以求均值
df4=clean_data(df4)
for i in df3.index:
start_date=i-np.timedelta64(days+1,"D")
ave=df4[(df4.index>start_date) & (df4.index<i)]['收盤價'].mean()
average.append(ave)
########求振幅#######
start2=df.head(1).index-np.timedelta64(wave+1,"D")
start2=start2.astype('str')[0].replace("-","")#轉換為字符串
df5=get_data('0000300',start2,'20191231')
df5=clean_data(df5)
for i in df3.index:
start_date=i-np.timedelta64(wave+1,"D")
interval=df5[(df5.index>start_date) & (df5.index<i)]['收盤價']
length=interval.max()/interval.min()-1#最大漲跌幅
wavelength.append(length)
return average,wavelength
智能定投策略計算收益率
def stratege(ml,wl,T_1):
'''
定投策略
:param ml:均線
:param wl:振幅
:param T_1:前1日收盤價
'''
cal=T_1/ml-1#大於0,則高於均線
if(cal>=0 and cal<0.15):
return 0.9
elif(cal>0.15 and cal<0.5):
return 0.8
elif(cal>=0.5 and cal<1):
return 0.7
elif(cal>=1):
return 0.6
elif(wl>0.05):
if(cal>=-0.05 and cal<0):
return 0.6
elif(cal>=-0.1 and cal<-0.05):
return 0.7
elif(cal>=-0.2 and cal<-0.1):
return 0.8
elif(cal>=-0.3 and cal<-0.2):
return 0.9
elif(cal>=-0.4 and cal<-0.3):
return 1.0
elif(cal<-0.4):
return 1.1
else:
if(cal>=-0.05 and cal<0):
return 1.8
elif(cal>=-0.1 and cal<-0.05):
return 1.9
elif(cal>=-0.2 and cal<-0.1):
return 2.0
elif(cal>=-0.3 and cal<-0.2):
return 2.1
elif(cal>=-0.4 and cal<-0.3):
return 2.2
elif(cal<-0.4):
return 2.3
完整策略實現
def smart_invest(df1,frequence,invest_money,start_time,days,wave):
'''
定投計算
:param df1: 數據集
:param frequence: 定投頻率
:param invest_money: 每次定投金額
:param start: 定投起始日期
:param days: 參考均線天數
:return (amount,invest_log): (收益數據DataFrame,定投記錄dict)
'''
invest_log={}#每次定投的日期記錄(日期:大盤指數)
invest_day=start_time#每次投資的時間
invest_amount=0#總投資金額
profile=0#總投資收益
amount=0#賬戶總資產
profile_log=[]#總收益日志
invest_amount_log=[]#賬戶投資金額日志
amount_log=[]#總資產日志
Yield=[]#收益率日志
df1["均線"]=mean_days(df1,days,wave)[0]#獲取均線
df1["振幅"]=mean_days(df1,days,wave)[1]#獲取振幅
for date,quote_change,index,ml,wl in zip(df1.index,df1['漲跌幅'],df1['收盤價'],df1["均線"],df1["振幅"]):
profile+=quote_change*amount#計算當天收益率
profile_log.append(profile)
#判斷是否為定投日
if date==invest_day:
if(invest_day==start_time):
T_1=start_time
else:
formal_day=1
while(True):
T_1=date-np.timedelta64(formal_day,"D")#前1天的收盤價
if(T_1 in df1.index.tolist()):
break
else:
formal_day+=1
T_1=df1[df1.index==T_1]["收盤價"][0]
rate=stratege(ml,wl,T_1)
invest_amount+=invest_money*rate#定投
invest_log[invest_day]=index#記錄定投當日的指數
#判斷7天后是否為交易日,如果不是則往后加1天直到找到交易日
invest_day+=np.timedelta64(frequence,'D')
flag=0
while(True):
if(df1[df1.index==invest_day].index==invest_day):
break
else:
invest_day+=np.timedelta64(1,'D')
flag+=1
if(flag==100):
break
invest_amount_log.append(invest_amount)
amount=invest_amount+profile#更新賬戶總資產
amount_log.append(amount)
try:
Yield.append(profile/invest_amount*100)#更新收益率
except:
Yield.append(0)
print("總投資:",invest_amount)
print("總收益:",profile)
print("收益率: ",profile/invest_amount*100,"%")
over=pd.DataFrame({
"日期":df1.index,
"收益率":Yield,
"賬戶資產":amount_log,
"投資金額":invest_amount_log
})
over=over.set_index("日期")
return over,invest_log
四、可視化普通定投與智能定投策略收益率
frequence=30#定投頻率
invest_money=5000#每次定投金額
start=np.datetime64("2018-01-02")
print("優化后的定投策略(參考500日均線,近5日振幅的月定投):")
res1,buy1=smart_invest(df3,frequence,invest_money,start,500,5)
print("======================================\n普通定投策略:")
res2,buy2=invest(df3,frequence,invest_money,start)
myplot(df3,res1,buy1,"2018-2019年滬深300 優化定投 收益率")
myplot(df3,res2,buy2,"2018-2019年滬深300 普通定投 收益率")
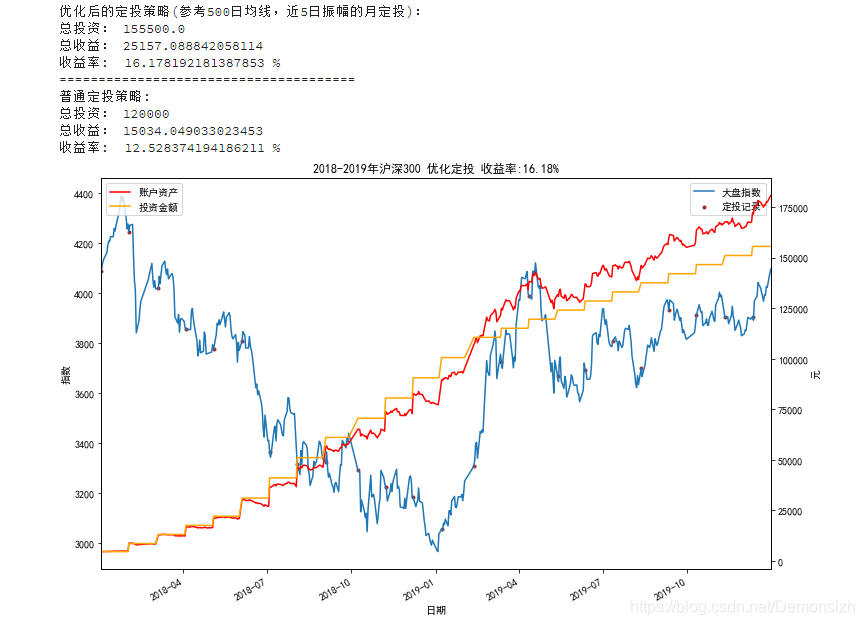
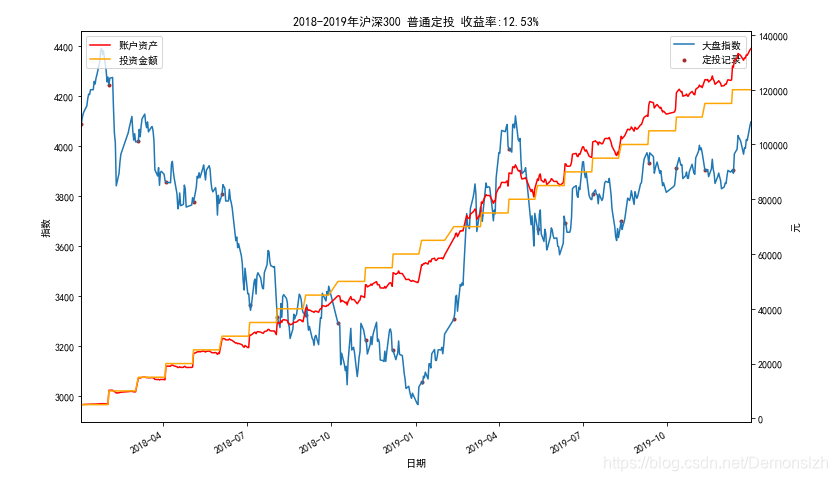
五、文末福利(完整代碼)
關注以下公眾號回復"0003"獲取完整源碼
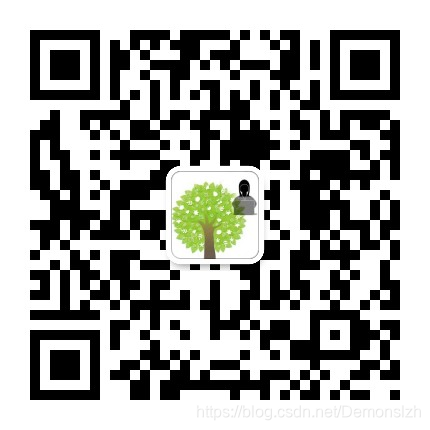